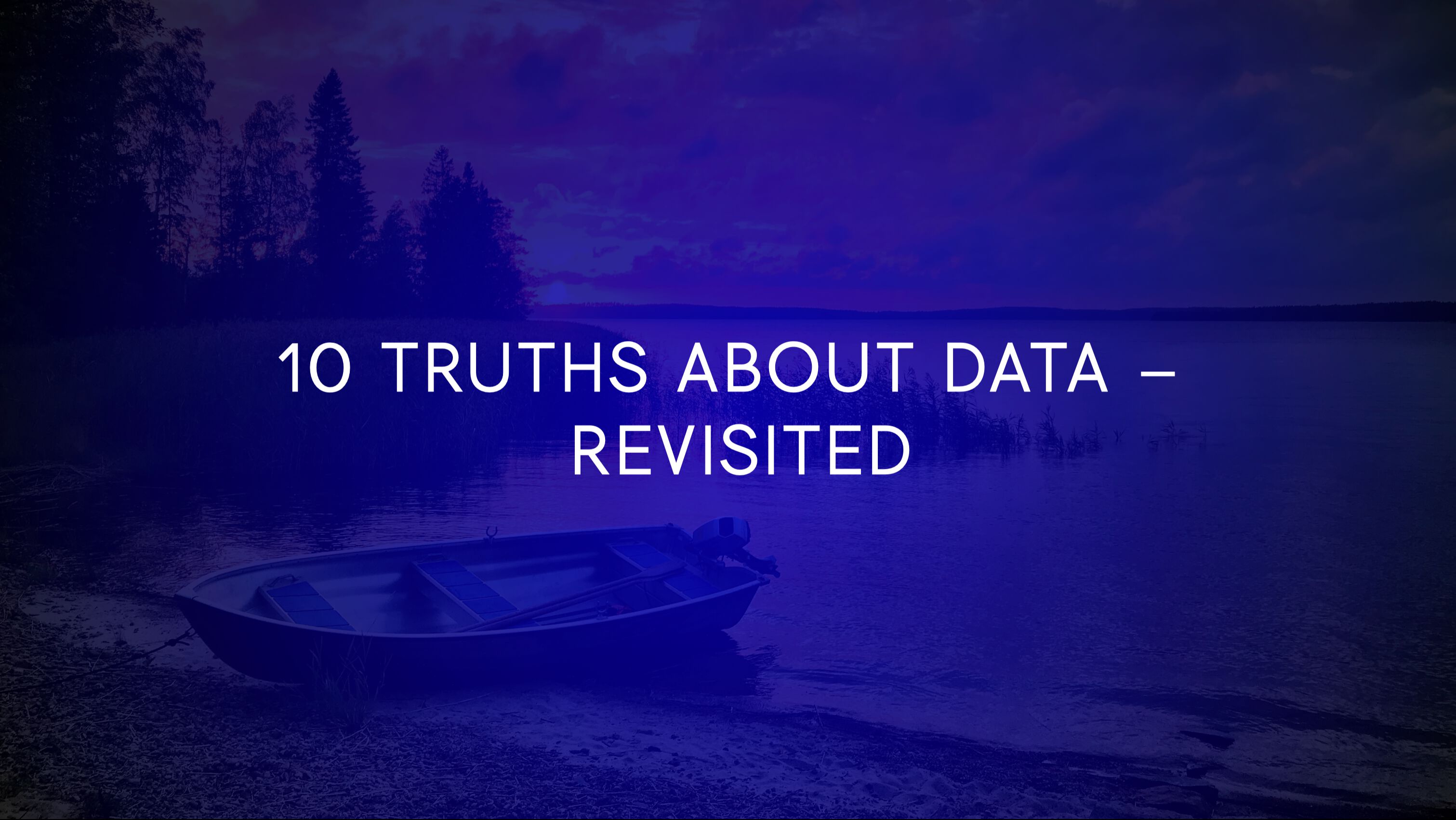
10 Truths About Data - Revisited
Seven years ago, I wrote on my former employer’s (the amazing Reaktor) blog a tongue-in-the-cheek article titled 10 Truths About Data.
Looking back on it today, I’m still proud of the handiwork, but I can’t help but think that some of the truths were wasted just to reach the magic number 10.
So, today, I want to revisit these truths and provide a rehashed version for you, my dear reader.
You might cynically think that this article is just a way for me to prevent having the first month in this blog’s history with no new articles published. And you would be partially right.
You might also cynically scoff at the notion of “truth” being tossed around in a cavalier manner. And you would be right in your indignation. However, “truth” has a nicer twang to it than “assertion” or “claim”.
Anyway. Let’s get to it.
XThe Simmer Newsletter
Subscribe to the Simmer newsletter to get the latest news and content from Simo Ahava into your email inbox!
Truth #1: Data is passive.
One of the OG truths.
Often, when presenting data, people use a phrase like “The data shows that…" or “The data clearly states that…". While I know what these people are trying to say, it’s still a semantic cop-out.
The data doesn’t do anything. It’s a passive medium – exploited, wrangled, manipulated, molded, and shaped to provide evidence or justification for, or even a diversion from, whatever the presenter is trying to state.
Truth #2: Data is subjective.
Another rehash from the first version of this article, and another no-brainer.
When you look at an analysis, or a graph, or the rows in a raw data table, you are generating a unique interpretation of what you see. There are no objective truths in the evidence in front of you.
This can easily slip into an ontological argument, and that’s fine. The fact is that data quality and analysis are not fixed. As I wrote in the original piece:
A single data set can shift from useless to incredibly insightful without a single datum changing shape, size, form, or function.
Truth #3: Data is boundless.
Oh yes – and the importance of this truth just increases as the scale of what we can and do collect increases in orders of magnitude with every passing year of technological advancement.
It’s impossible to have all the data. It’s not just technologically infeasible – it’s a philosophical impossibility.
So a line must be drawn. And it’s so very, very important to understand where this line is plotted. You must understand the limitations of your data set when offering it as evidence with any sort of representational capability. You must be able to communicate these limitations when prompted for, or even proactively in order to keep the results fair and reproducible.
Truth #4: Data hates silos.
OK, I used the word “abhors” in the first version of this list, but that was just a Thesaurus talking.
For some baffling reason, many companies still treat data as something that can be delegated to an arbitrary job title (the analyst or the data engineer or the scientist) while the rest of the company proceeds to ignore (and neglect) the all-encompassing reach of the data pipeline.
Data is the lifeblood of the organization. It doesn’t care about job titles. It doesn’t care about your matrix organization or your flat hierarchies or your unlimited PTOs.
You need to know all the nooks and crannies within your company where data is being collected and processed, and you need to constantly evaluate and audit these processes.
Truth #5: Data is a process.
Picking up from above – remember that data isn’t something you can just wrap up in a one-off project. From a regulatory point of view, your company has a responsibility of being in touch with the upstream and downstream impact of all the data wrangling going on within (and beyond) its walls.
But it’s not just that. Your company is generating absurd amounts of data with every passing second. You need to react to fluctuations thereof (and things are constantly in flux), and you need a process in place to properly nurture the data pipelines within your company.
Truth #6: Data can be ignored.
My favorite truth.
Being “data-driven” is a lie! Don’t fall for it! Based on some 20 years of experience, most companies work with data that is completely misunderstood and where the baseline quality is just ridiculously poor (although, remember Truth #2!).
If you want that smelly heap of ones and zeros to drive your company then be my guest. Wave to me while plunging into the abyss with a data-driven smile on your face.
If the data says A, and this is backed by experimentation, rigorous testing, and as solid a data set as you will ever come across, but your gut says B, feel free to go with the latter! You can ignore the data. There is no categorical imperative compelling you to do what the data says (although, remember Truth #1!).
However. However. You must be able to justify this so that the business case makes as much or more sense as going with what the data analysis instructs you to do.
You can’t just throw a hissy fit and ignore the data because you feel it is your divine right to walk off the edge of the Earth just to prove a belabored point. You need to be able to build a business case for your decision, and you need to be able to convince your colleagues that it’s worth the risk.
Truth #7: Tools can’t dictate how your organization works.
For some reason, many data platforms are very prescriptive. They force the company to adopt schemas that might not be relevant to the business cases of the company but instead only serve to make the analytics platform digest the information in a predictable way.
Monolithic, generic schemas are, in general, a bad thing. They force the company to adjust to the analytics platform rather than the other way around.
I remember spending many a second wondering just how I can “cheat” Google Analytics to digest an Add To Cart event on a website that didn’t have a shopping cart; just so that I could use the ecommerce report suite. This is an exercise no one should have to endure.
Truth #8: Real insights are rare, and that’s OK.
I feel like many analysts act like John Nash in A Beautiful Mind, where they look at a data set and hope that patterns will just jump out, fuelling some amazing new insight that will completely turn their company around.
Well, you’re either in for a long wait or you’re not doing your job well.
There’s a lovely theory in evolutionary biology called punctuated equilibrium. It states that most of evolution is actually a very slow, steady progress. However, occasionally momentous upheavals happen, introducing chaotic, more rapid change in the process.
I’m not an evolutionary biologist, but this theory was adopted into linguistics by R.M.W. Dixon, which is a genre I’m much more familiar with.
I think that many analysts don’t respect this, and they try to either find these upheavals unsuccessfully or, worse, they try to introduce them with new tools, new collection methods, and new schemas, just to “get results”.
But the fact is that much of what we do in analytics is based on steady observation and providing stable data for other processes to digest.
We are gardeners. Not treasure hunters.
Truth #9: Data is a side effect.
OK, this isn’t always true (shocking!), but it’s particularly poignant in the world of digital marketing and analytics.
There are very, very few actual features in apps, sites, and services whose main purpose is to generate data.
Instead, as analysts, we most often tap into existing features, and add data collection as a side effect to them.
The main purpose of a checkout form isn’t to generate a conversion. No – its main purpose is to generate a purchase. The conversion ping is just a side effect of this process.
As analysts, we tend to get caught up in the importance of our work, and we forget that most of the time our companies, our clients, our developers, or even our marketers don’t care that much about the data generation. They just want the feature to serve its original purpose.
For this reason, data engineering tasks are often deprioritized. It’s a shame, but it’s also a fact.
The person working with the data needs to clarify the importance of these side effects, too. The role of the data engineer (or analyst) is often one of consultation, as they need to make others understand how these side effects can actually be worth the investment of time and resources rather than just development overhead.
Truth #10: Data is difficult.
For years and years, all my presentations ended with a slide that said:
Data is difficult. Data quality is earned, not acquired.
This, I think, is still very important. Particularly with the COVID-19 pandemic, more and more people were exposed to more and more charts, more and more analyses, and more and more misguided interpretations of data.
I hope people understand how difficult it is not only to collect data, but to figure out its processing flows, its downstream impacts, its regulatory challenges, and how to present it in a meaningful way.
I hope people understand that “ML” and “AI” aren’t just magical buzzwords. Algorithms that fuel machine learning and artificial intelligence require fine-tuning and a human component with enough expertise (and courage) to set the processes in motion.
Working with data is as difficult as it has ever been. There still are no shortcuts: data quality must be earned through hard work, with a curious mind, and a strong heart.
Simo out.